It’s almost impossible to have a business conversation without a mention of AI — and for good reason. Generative AI can help make our work days more efficient, sometimes at the click of a mouse or a simple ask of a question. The more you explore the possibilities of AI, the more you will be met with terms that may be new or unfamiliar. The key to superior AI results is knowing both how to use the technology and what to do with the information output. To help make your digital journey more accessible, here are the top 16 generative AI terms you should know.
Artificial intelligence is the field of study that’s attempting to replicate human intelligence using computer systems. We break that down to trying to get computers to understand, learn, reason, and interact the way humans do. It’s a broad term that covers far more technologies than ChatGPT. Other areas include: computer vision, speech recognition, robotics, expert systems, and more.
Machine learning is a technical breakthrough that is applicable across different domains of AI. Machine learning enabled computer systems to “learn” the way humans do. Now they no longer have to be specifically programmed to do a specific task, they can “learn” based on observing large amounts of correct input/output pairs.
Natural language processing is what enables a computer to “understand” the meaning of a sentence. It does this by parsing the sentence first into nouns, verbs, etc.. and then using grammar rules to understand meaning and intent. Sometimes it is also referred to as NLU (natural language understanding).
Chatbot is the friendly word for a program that imitates having a conversation, it is a more personified name. It does NOT have to have AI as the technology behind it. Early versions of chatbots only had a conversation tree behind it. If you remember the bank call systems of press 1 for accounts, and press 2 for balances, you understand exactly how a conversation tree works. It’s only recently that Chatbots changed the technology to be AI driven.
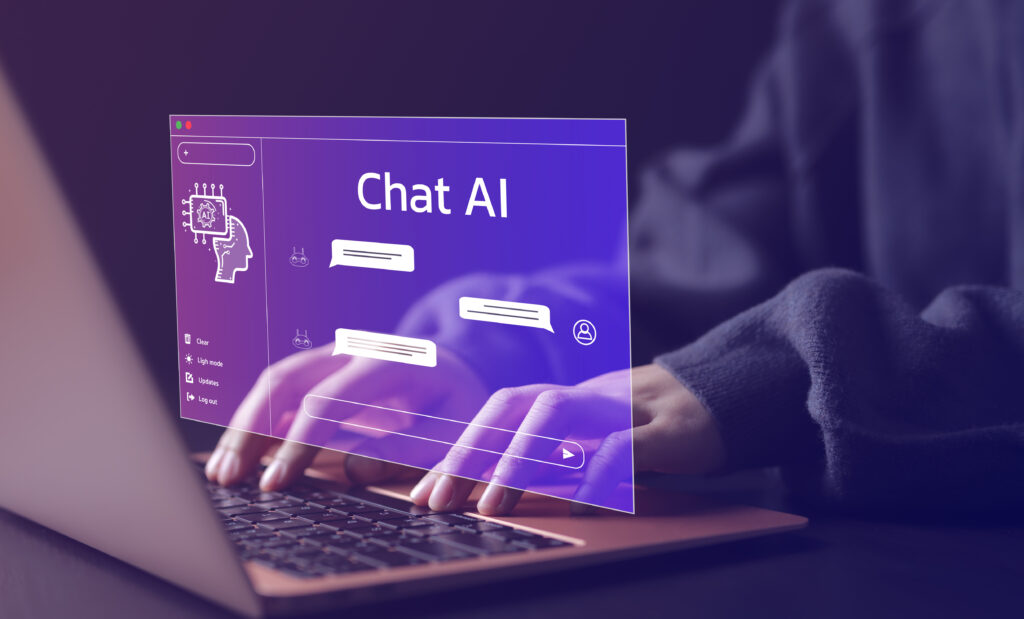
Generative Pre-trained Transformer is GPT. Transformer is a machine learning technique that increases the efficiency of ML tremendously. That’s why ChatGPT is able to be trained on 10% of the internet in a few months time. The P in GPT is for pre-trained, this is why ChatGPT has a knowledge cutoff time because it’s pre-trained only by data up till April 2023 (for the new GPT 4). The G refers to the generative nature of the model, which essentially is what allows ChatGPT to “generate” responses based on questions.
Fine-tuning is essentially an adjustment on a very large model to be more specific in knowledge or behavior. A very common fine-tuning use case is for domain-specific vocabulary and knowledge. $AAPL for example is used by traders to refer to the technology company Apple.
ChatGPT, Bard, and Claude are all conversational AI. This term specifically refers to chatbots driven by AI.
AI ethics is now the umbrella term that covers responsible AI, observability in AI, AI legal and compliance risk, and AI bias.
Bias in AI refers to the fact that the large language models today are trained on essentially data and text that’s been crawled from the internet, utilizing western media in particular — and a large amount of that information is social media and advertising. Since the models are created using machine learning, they essentially learn from the most common patterns that they see. If most CEOs on social media and the internet are men, the model will have a bias that, in general, CEOs are men. Because the required dataset is so large to train AI models, it’s difficult to carefully assemble a complete data set for AI model training outside of crawling the web. However, companies are starting to address the problem through other techniques.
Because of all the potential issues in AI ethics and the unpredictable behaviors of generative AI, the only way to ensure output quality is to put a human-in-the-loop (HITL). Which is just basically: proofreading AI-generated content before you send it out.
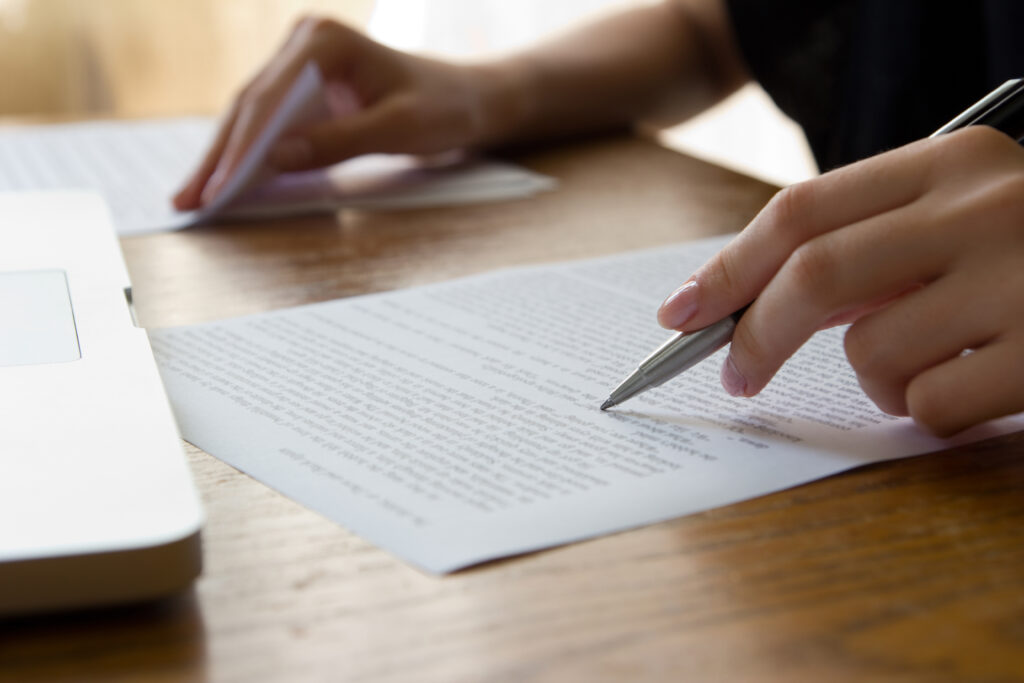
The questions and tasks you ask of ChatGPT, Bard, and Claude are called prompts. There are different ways to write long, complicated, structured prompts to control the responses you get back. This practice is called prompt engineering. Contrary to what it looks like, it does NOT involve actual engineering and you do NOT need technical skills to be good at it.
The simplest way to think about the context window of a chatbot or LLM (large language model) is memory. The longer the context window is, the longer the chatbot remembers what you have said. The context window includes both what you say and what the chatbot responds with. So even if you asked a short question, but the chatbot response is very long, it will stop remembering what you said much quicker.
The results of fine-tuning is very often a domain-specific LLM; CodeLlama is a good example. It’s specifically fine-tuned on code. There’s also Harvey, a legal LLM.
Multi-modal AI is discussed a lot because traditionally AI models are single-modal only. The image model is completely separate from a text model, and a language-based model is separate from a music-based model. A true multi-modal AI is a single model that can understand and generate multiple modes like text, image, and sound.
Computer systems have had two separate approaches to generating human conversation, the old way was to use grammar rules to compose correct sentences. While not technically wrong, comes across awkwardly. The new generative conversation AI however, uses human mimicry, which means it’s simply trying to replicate speech patterns that are in the training data — which in this case is a lot and lot of X, formerly Twitter, threads. While this produces much more realistic sounding human conversations, it also introduces AI bias.
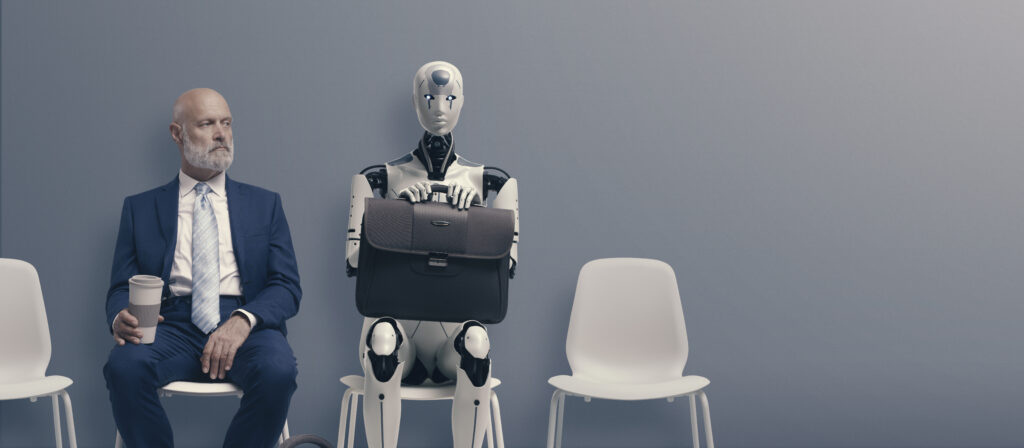
Knowledge integration is just a fancy term for uploading files. Once that knowledge is uploaded, you can ask the AI you are using to answer based on the information in those files. With the latest functionality, that file can be a local file, or it can be a URL. Now you’re no longer limited to the data that is pre-trained in the model with this addition of knowledge integration.
Now that you know and understand these terms, you’re well on your way to becoming a generative AI authority. Browse the PEAK6 portfolio of companies to see what we’ve been up to with AI and check our open positions if you’re interested in joining us on the forefront of AI.